Combining Human Expertise and Machine Learning for Intelligent Transportation Resource Management
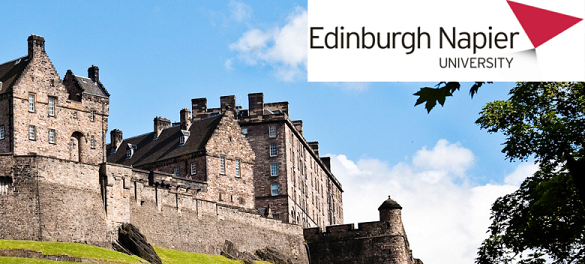
Typically, logistics networks generate billions of new data items on a monthly basis that can be used to help predict potential customer behaviour. Lorries transport thousands of pallets on hundreds of trailers for millions of customers scattered across hundreds of thousands of postcodes. Customers are continuously placing orders with information being generated about what they want to transport, where it will be coming from, where it will be going and what their service requirements are. All these orders need plans for carrying the pallets associated with them, with varying time horizons, depending on (1) when the information becomes available and (2) whether they lead to urgent additional capacity needs.